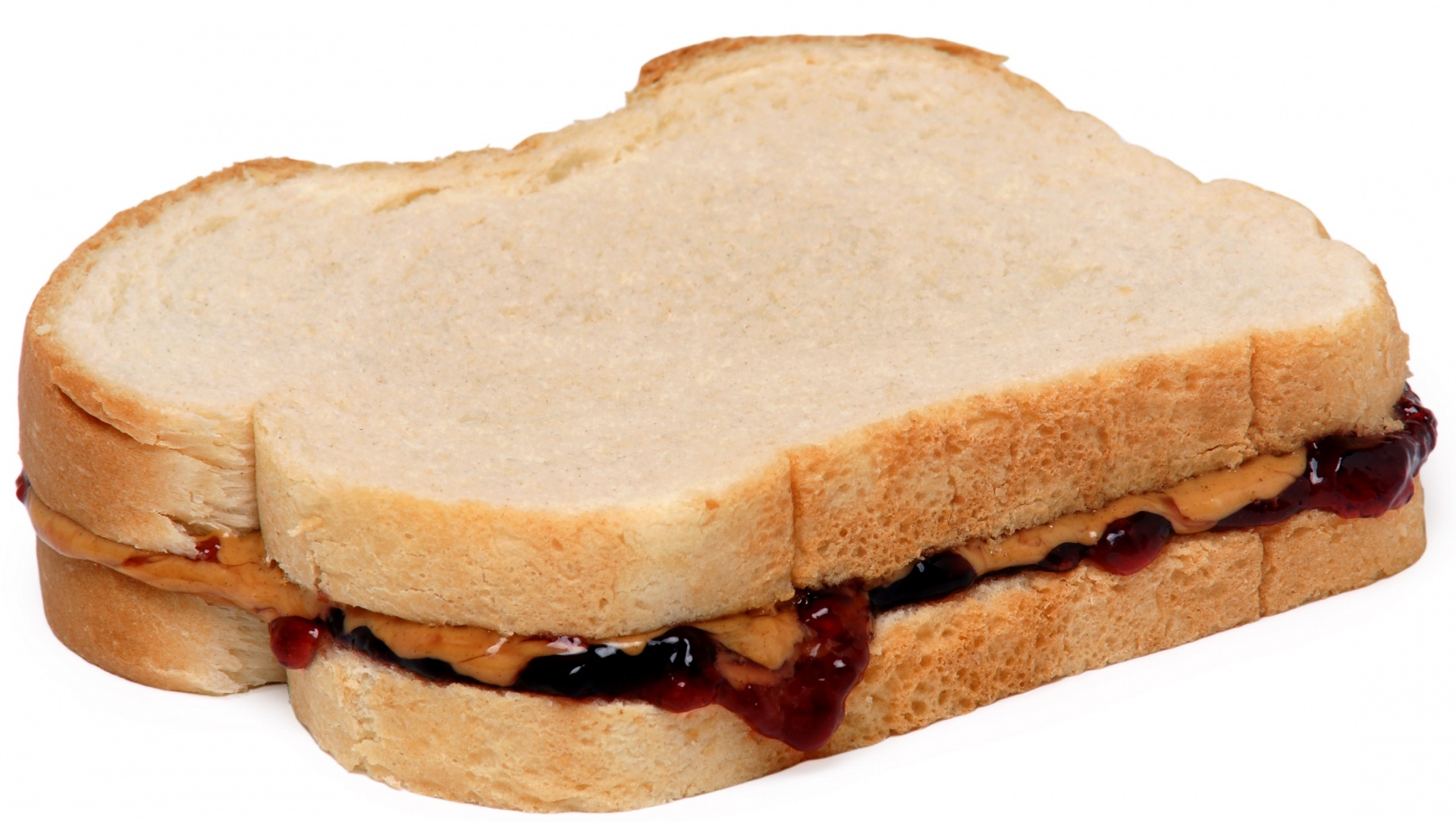
Principal Components Analysis – and everyone’s favorite sandwich!
Is your survey scale or instrument measuring one, and only one psychological construct? How do you know? What does it matter?
As our Data Scientist, David Meier will tell you, refining your survey scales to ensure they are measuring unique constructs is critical for the validity of your inferential statistical interpretations. You can’t truly know if someone likes peanut butter or jelly more if your scale is asking them about peanut butter and jelly simultaneously. That’s where dimension reduction techniques come in. Dimension reduction techniques help you discover how many “dimensions” are being captured by your survey scale questions based on the responses of your participants. Using a dimension reduction technique, you could discover that your peanut butter and jelly questions really have two dimensions, a “peanut butter liking” dimension and a “jelly liking” dimension. Armed with this knowledge, you can put the really “peanut buttery” questions into their own scale, the really “jelly” ones into theirs, and cut out the ones that don’t seem to capture more of one than the other.
There are several dimension reduction techniques out there, but Dave’s “go-to” is Principal Components Analysis (PCA). While more specialized and complex dimension reduction techniques exist, PCA is relatively straightforward and a great place to start your dimension reduction efforts. Below are three links to works that employed PCA with regard to Student Attitudes Toward STEM Education, STEM Interest, and STEM Career Interest, one of which even co-authored by our very own Lynn Dierking and John Falk!
JOTS v36n1 – Complete Issue (ed.gov)
Tyler-Wood et al (2010).pdf (edc.org)
Posted May 12, 2021